With the rapid digitalization of business processes, companies are recognizing the importance of aligning their data management strategy with their organizational goals.
This alignment is essential for optimizing business operations, leveraging real-time analytics, driving cultural change, and handling compliance more effectively.
Data management trends in 2024 highlight the shift towards digitized business models that stand out through solid data management practices across operations.
Organizations that need to implement an impactful data management strategy encounter inefficiencies in handling daily tasks due to duplicated data and data management gaps across departments.
McKinsey’s research finds only 30% of companies successfully avoid these pitfalls by coordinating their organizational strategies with their data strategies.
This coordination is pivotal for optimizing business processes, removing redundancies, eliminating unnecessary manual efforts, and enhancing revenue growth.
Let’s analyze some data management strategies and determine how they can improve your business.
Definition of Data Management Strategy
Data management strategy is an overall vision and approach for the management of an organization’s data, taking into account the strategic objectives, business processes, information governance principles, and technological context.
This strategy encompasses collecting, organizing, protecting, and storing an organization’s data so it can be analyzed for business decisions.
The significance of a well-defined data management strategy lies in its ability to handle and utilize data effectively.
With proper data management, your business can make decisions based on stale or accurate data, which could be beneficial.
Effective data management ensures data is available, accurate, and well-secured throughout its lifecycle. It supports strategic decision-making to improve business outcomes.
Components of a Data Management Strategy
These components provide a unified foundation for managing how data is handled within your company and how it aligns with business processes and operations.
1. Data Governance
Data governance refers to the process of managing the availability, integrity, security, and usability of data in enterprise systems. It involves establishing internal standards and policies that guide data usage.
Effective data governance ensures that data remains accurate and consistent and is used effectively.
It plays a crucial role in managing data quality and integrity by enforcing standards that make data reliable and valuable for decision-making.
Key principles include defining clear roles and responsibilities, ensuring data quality, and complying with relevant regulations.
Practices involve setting up a data governance framework, regular audits, and aligning data use with business objectives.
A strong data governance program safeguards against data breaches and supports organizational goals by enabling reliable data.
2. Data Architecture
This data architecture outlines how data is processed, stored, and utilized within an organization, ensuring that data structures are managed efficiently.
Components like databases, data models, and schemas are integral to data architecture.
They help in organizing data effectively, making it accessible and useful for business processes. Databases store data, data models outline how data is related, and schemas define the organization of data in databases.
This structure supports the integrity and usability of data, facilitating better decision-making and operational efficiency.
The importance of data architecture extends to supporting scalability and flexibility in data operations, enabling businesses to adapt to changing data needs and technologies.
3. Data Quality Management
A robust data quality management strategy involves systematic processes and tools designed to maintain the accuracy, consistency, and reliability of data across its lifecycle.
The crux lies in implementing comprehensive data quality tools that automate the detection and rectification of data anomalies.
These tools are adept at cleansing, matching, and profiling data, thus ensuring that the data adheres to predefined standards and formats.
Moreover, the process encompasses regular audits and validations to safeguard against data degradation over time.
Such diligence not only enhances decision-making but also bolsters regulatory compliance, reducing the risk of data-driven inaccuracies that could lead to significant operational setbacks.
4. Data Security
Data security stands as a critical pillar in a data management strategy, focusing on safeguarding sensitive information from unauthorized access and breaches.
This aspect of data management employs a multifaceted approach, incorporating encryption, robust access controls, and a variety of security measures tailored to protect data integrity and confidentiality.
Encryption serves as a formidable barrier, transforming data into unreadable formats for unauthorized users.
At the same time, access controls ensure that only authorized personnel have access to sensitive information based on their roles and necessities.
Additionally, the implementation of comprehensive security protocols, such as intrusion detection systems and regular security audits, plays a crucial role in preempting potential vulnerabilities and threats.
Through such stringent security measures, organizations can significantly mitigate the risk of data breaches, thus preserving trust and compliance in the digital age.
Types of Data Management Strategies
Each type of data management below offers distinct advantages and challenges, with the optimal strategy often lying in a balanced, thoughtful combination of elements from each model to meet the unique data management needs of the business.
1. Centralized Data Management
Centralized data management amalgamates data into a singular repository, such as a data warehouse or lake, promoting consistency, accuracy, and ease of access.
This model facilitates better data analytics by ensuring that all relevant data is readily available for queries, thereby producing accurate, actionable insights.
It enhances operational efficiency through cost-effective, scalable data storage solutions. It decreases the maintenance burden by leveraging cloud-based services for data management.
Centralized systems also offer increased resilience against data loss and enable better data shareability within an organization and with external partners.
Industries like e-commerce, entertainment, and customer relationship management significantly benefit from centralization due to the streamlined analysis and personalized customer experiences it enables, as seen in companies like Amazon, Netflix, and Salesforce.
Centralization can introduce a single point of failure, raising privacy concerns and necessitating robust data protection protocols to mitigate risks associated with centralized repositories.
2. Decentralized Data Management
Decentralized data management distributes data across various locations or systems, enhancing data ownership, scalability, and fault tolerance.
This approach allows individual departments or teams to tailor data management practices to their specific needs, fostering innovation and agility within organizations.
The decentralized model’s inherent scalability supports business expansion without the infrastructure limitations of centralized systems, and its resilience ensures business continuity even when part of the system fails.
However, addressing challenges such as maintaining data consistency, complex data integration, and increased security risks due to data being spread across multiple nodes is crucial.
Decentralized strategies require robust synchronization, interoperability, and security measures to ensure data integrity and secure access.
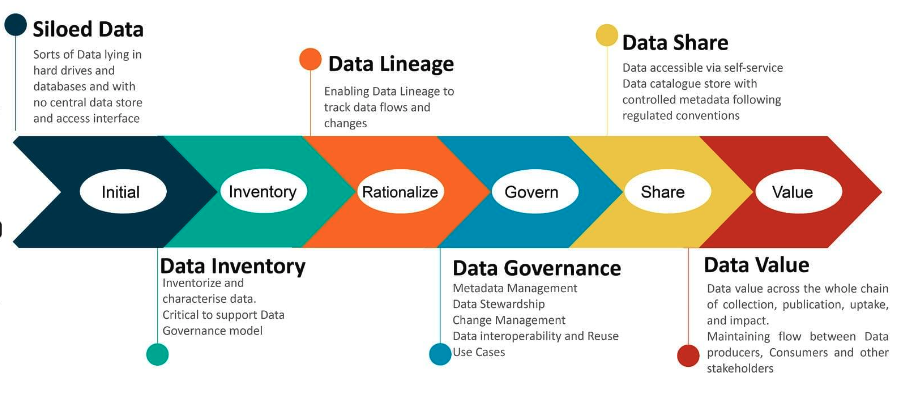
3. Hybrid Data Management
Hybrid data management combines centralized and decentralized approaches, aiming to leverage the benefits of both strategies while mitigating their respective drawbacks.
This model allows for centralized control over critical data assets for consistency and compliance while also distributing other data sets to foster agility and innovation where it benefits the organization most.
A successful hybrid strategy often involves utilizing centralized data warehouses or lakes for core business analytics and operational data.
This strategy is enhanced by decentralized databases or cloud services for department-specific, high-velocity, or experimental projects.
An example of a successful hybrid data management strategy is Hybrid Transaction/Analytical Processing (HTAP) by Gartner.
HTAP architectures enable the simultaneous execution of transactional and analytical processes, breaking traditional barriers between these operations.
This model provides businesses with real-time decision-making capabilities, enhancing online decision-making and software design flexibility.
How to Create a Data Management Strategy
These elements work together to drive growth opportunities and competitive advantage. They make your business agile and responsive to market changes.
1. Step 1: Assessing Current Data State
Assessment begins with understanding the current data landscape and evaluating existing data management practices, tools, and infrastructures.
You can utilize some tools like Talend Data Quality, Informatica Data Quality, IBM InfoSphere Information Analyzer, and SAS Data Management.
Some methodologies such as SWOT (Strengths, Weaknesses, Opportunities, Threats), data quality assessment, data maturity model, and data governance framework assessment.
These tools and methodologies are essential in identifying the strengths and gaps in the current system.
This assessment should uncover not only technical deficiencies but also strategic opportunities for leveraging data in areas previously unexploited.
Identifying your business’s specific data management weaknesses and strengths can help shape your strategy to address critical gaps.
At the same time, this step will leverage your unique data assets to ensure compliance and increase the strategic value of your data.
2. Step 2: Defining Goals and Objectives
Goals should not only be clear and actionable but also intricately linked to your business growth objectives.
For instance, enhancing customer experience might involve integrating real-time data analytics to personalize services, thereby directly boosting customer satisfaction and loyalty.
The focus on improving operational efficiency could be on automating data processes, significantly reducing manual errors, and accelerating decision-making.
Regulatory compliance could mean deploying advanced data governance tools to mitigate risk and build trust with stakeholders.
With such focused goals, the data management strategy becomes a critical foundation for innovation, driving business growth through improved decision-making, customer insights, and operational excellence.
This strategic alignment ensures that every data initiative contributes directly to the company’s competitive advantage and market responsiveness.
3. Step 3: Designing the Strategy
Designing a comprehensive data management strategy requires a systematic approach to integrate data with business goals effectively.
Begin by identifying your enterprise’s current requirements and objectives to ensure your data strategy supports business outcomes directly.
A critical aspect involves conducting a data discovery process to gain insights into data locations, purposes, and how it aligns with business needs.
This process should include an evaluation of both internal and external data sources to understand the marketplace better.
Furthermore, coordinating business units to standardize data processes without disrupting their operational value is essential.
Establishing a unified data governance model is crucial to managing data access, ensuring security, and complying with privacy regulations.
Finally, defining measurable metrics and a Data Strategy roadmap facilitates the transformation of a business by clearly communicating how strategic objectives will be met through data management.
4. Step 4: Implementation and Monitoring
In the implementation phase, it is vital to select the right technology that aligns with your data management processes, from data collection to analysis and distribution.
This ensures that data activities are as effective and efficient as possible.
Tableau, for example, offers a data management add-on to help manage analytics environments, streamline processes, and ensure that data-driven decisions are based on up-to-date and trusted data.
Establishing robust data governance is another cornerstone, setting the stage for responsible data use across the organization.
Data governance frameworks should address data quality, security, privacy, and ethical use to ensure that data benefits the organization without introducing risks.
Training and skill development are equally critical, ensuring that all stakeholders understand their role in the data management strategy and are equipped to execute it effectively.
This includes providing tools and knowledge to departments beyond IT fostering a data-centric culture throughout the organization.
Conclusion
So, here’s the deal: we all get that a smart data management strategy can seriously boost your business. But, let’s be real, it comes with its fair share of headaches.
Especially for small businesses, it feels like wading through a swamp of confusion and chaos.
Why? Mostly because of the tight resources. These strategies sometimes play poorly with the realities of running a small operation.
In today’s fast-moving business world, lots of companies are hitting snags, especially with making their data work for them efficiently.
Therefore, Nexalab’s Data Visualization and ETL services offer a transformative approach to managing your business data.
If you’re running a business in Australia and drowning in data woes, check out our services. Here’s the scoop: we take your data from all over the place, clean it up, make sure it’s spot-on, and then turn it into something you can actually use to make smart decisions.
Nexalab hooks you up with a one-stop shop for all your data needs, making your life a whole lot easier.
Can we level up your data management strategy? Dive into Nexalab’s Data Visualization and ETL services and see for yourself.